In Silico
Leveraging In Silico Computational Chemistry Across Scientific Disciplines.
In the modern scientific landscape, in silico computational chemistry has emerged as a powerful tool that transcends traditional disciplinary boundaries. This virtual laboratory environment offers researchers from diverse fields the ability to model, simulate, and analyze complex chemical systems without the need for physical experiments. Let’s explore how different types of scientists can harness this technology to advance their research, particularly focusing on the capabilities offered by the AlphaPerrin computational chemistry environment integrated with Gaussian software.
Inorganic Chemists:
Inorganic chemists can use computational methods to predict the structure and properties of novel metal complexes. This involves using quantum mechanical calculations to determine the most stable geometries, bond lengths, and electronic configurations of complexes that have not yet been synthesized. By doing so, researchers can screen potential compounds for desirable properties before investing time and resources in actual synthesis. In the AlphaPerrin environment, inorganic chemists can leverage Gaussian’s powerful DFT methods to optimize geometries and calculate electronic properties of metal complexes efficiently.
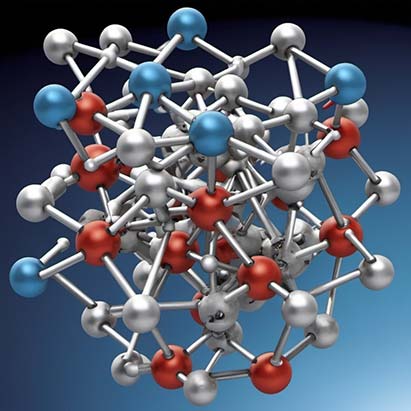
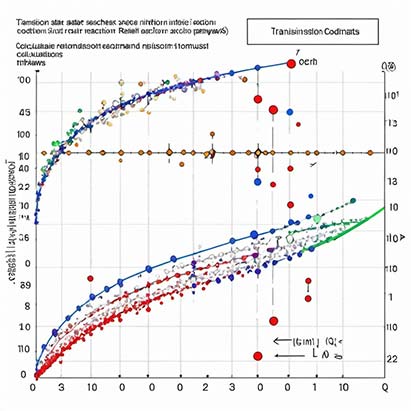
Studying reaction mechanisms involving transition metals is another key application. Computational tools allow inorganic chemists to model the step-by-step progression of reactions, identifying intermediates and transition states that may be too short-lived to observe experimentally. This can provide crucial insights into how catalysts work and how to design more efficient ones. Using AlphaPerrin’s integration with Gaussian, researchers can perform transition state searches and intrinsic reaction coordinate calculations to map out detailed reaction pathways.
Modeling catalytic processes in detail is particularly valuable for industrial applications. Researchers can simulate how different catalysts interact with reactants, how they lower activation energies, and how they influence product distributions. This can lead to the development of more efficient and selective catalysts for a wide range of industrial processes. The AlphaPerrin environment allows for seamless setup of complex catalytic systems, while Gaussian’s advanced solvation models can account for realistic reaction conditions.
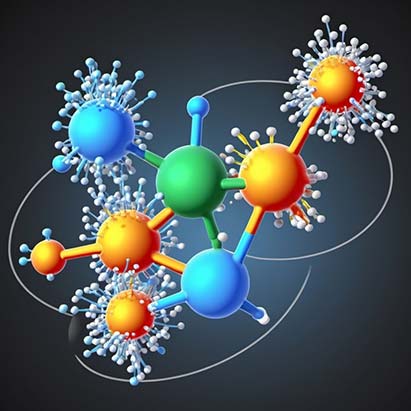
Investigating the electronic structure of organometallic compounds allows inorganic chemists to understand the nature of metal-carbon bonds and how they influence reactivity. Computational methods can reveal details about orbital interactions, electron density distributions, and bonding characteristics that are difficult to probe experimentally. AlphaPerrin’s visualization tools, coupled with Gaussian’s population analysis methods, enable researchers to gain deep insights into the electronic structure of organometallic compounds.
Physical Chemists:
For physical chemists, in silico tools enable the simulation of molecular dynamics and kinetics. These simulations can track the movement and interactions of molecules over time, providing insights into reaction rates, diffusion processes, and the behavior of materials under different conditions. This is particularly useful for understanding complex systems like proteins in solution or the behavior of gases in atmospheric chemistry. AlphaPerrin’s molecular dynamics modules, integrated with Gaussian’s force field parameterization capabilities, allow physical chemists to set up and run sophisticated MD simulations.
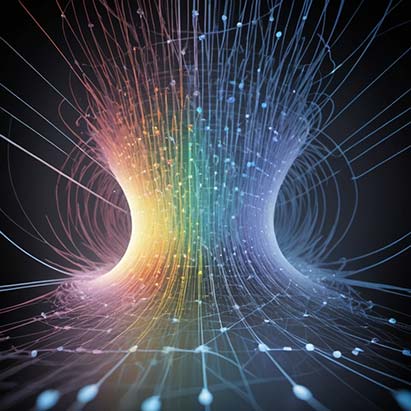
Modeling of spectroscopic properties is another powerful application. Computational methods can predict NMR spectra, vibrational frequencies, and electronic transitions, helping researchers interpret experimental data and design new spectroscopic experiments. This is especially valuable for studying transient species or compounds that are difficult to isolate. Gaussian’s extensive suite of spectroscopic prediction tools, accessible through the AlphaPerrin interface, enables accurate modeling of various spectroscopic properties.
The study of surface chemistry and adsorption phenomena benefits greatly from computational approaches. Physical chemists can model how molecules interact with surfaces, predicting adsorption energies, surface diffusion rates, and the impact of surface defects. This has applications in heterogeneous catalysis, materials science, and environmental chemistry. AlphaPerrin’s periodic boundary condition capabilities, combined with Gaussian’s surface chemistry modules, provide a powerful platform for studying surface phenomena.
Investigation of quantum effects in chemical systems is a frontier area where computational chemistry excels. Physical chemists can model phenomena like tunneling, zero-point energy, and quantum coherence, which are crucial for understanding processes ranging from enzyme catalysis to the operation of quantum computing devices. The AlphaPerrin environment offers access to Gaussian’s advanced quantum chemistry methods, allowing researchers to probe these subtle quantum effects with high accuracy.
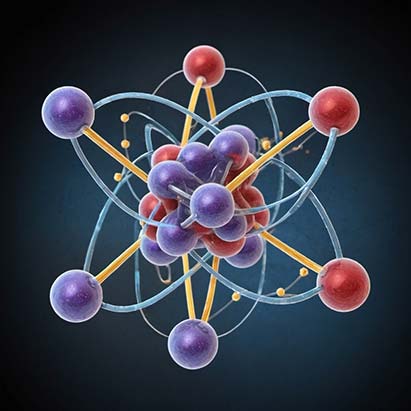
Theoretical Chemists:
Performing high-level quantum chemical calculations is a cornerstone of theoretical chemistry. These calculations can provide extremely accurate predictions of molecular properties, reaction energies, and spectroscopic observables. As computational power increases, theorists can tackle larger and more complex systems with higher levels of theory. AlphaPerrin’s integration with high-performance computing resources enables theoretical chemists to harness Gaussian’s most advanced and computationally intensive methods for studying large molecular systems.
Studying electron correlation effects is crucial for understanding many chemical phenomena. Theoretical chemists use computational tools to model how electrons interact and influence each other, going beyond simple mean-field approximations. This is essential for accurately describing bond breaking, excited states, and many-body effects in materials. Gaussian’s post-Hartree-Fock methods, accessible through AlphaPerrin, allow theoretical chemists to explore electron correlation effects with high accuracy.
Exploring potential energy surfaces of chemical reactions allows theoretical chemists to map out all possible pathways a reaction might take. By identifying minimum energy paths, transition states, and intermediates, they can predict reaction mechanisms, rate constants, and product distributions. This information is invaluable for understanding complex reactions and designing new synthetic strategies. AlphaPerrin’s automated workflow capabilities, combined with Gaussian’s transition state search algorithms, enable efficient exploration of complex potential energy surfaces.
Biochemists
Biochemists can leverage in silico computational chemistry to study the structure and function of biomolecules such as proteins, nucleic acids, and lipids. Using the AlphaPerrin environment, biochemists can perform advanced molecular modeling and simulation tasks to understand these complex biological systems at an atomic level. The integration with Gaussian software allows for high-accuracy quantum mechanical calculations, providing detailed insights into electronic structures and energetics of biochemical processes.
One key application for biochemists is the prediction and analysis of protein structures. AlphaPerrin’s protein modeling tools, coupled with Gaussian’s geometry optimization algorithms, enable researchers to predict the three-dimensional structures of proteins from their amino acid sequences. This is particularly valuable for studying proteins that are challenging to crystallize or too large for NMR analysis. Biochemists can use these computational models to investigate protein folding pathways, identify potential binding sites, and predict the effects of mutations on protein stability and function.
Enzyme catalysis is another area where computational methods excel in biochemistry. AlphaPerrin’s QM/MM (Quantum Mechanics/Molecular Mechanics) capabilities, integrated with Gaussian’s reaction path analysis tools, allow biochemists to model enzyme-catalyzed reactions with unprecedented detail. Researchers can simulate the entire reaction mechanism, including the formation and breaking of chemical bonds, while accounting for the protein environment. This approach helps elucidate the roles of specific amino acid residues in catalysis and guides the design of novel enzymes with enhanced activities or specificities.
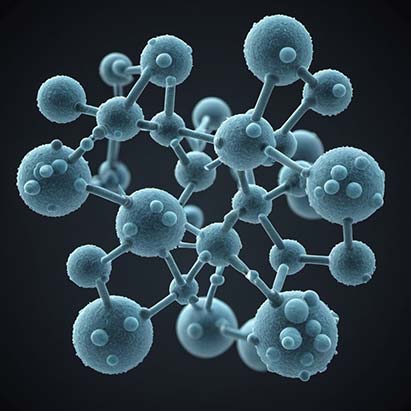
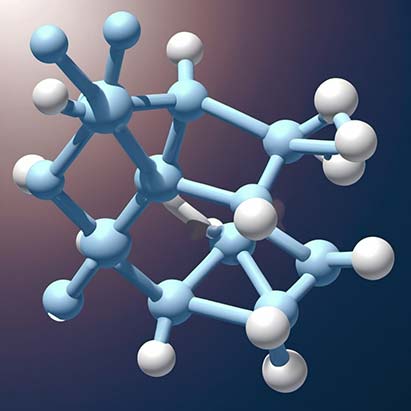
The study of protein-ligand interactions is crucial for drug discovery and understanding cellular signaling processes. AlphaPerrin’s molecular docking modules, combined with Gaussian’s binding energy calculations, provide biochemists with powerful tools to predict how small molecules or other proteins bind to target biomolecules. These computational methods can screen large libraries of compounds to identify potential drug candidates, optimize lead compounds for better binding affinity, and predict potential side effects by identifying off-target interactions.
Membrane protein studies, which are notoriously challenging experimentally, benefit greatly from computational approaches. AlphaPerrin’s membrane modeling capabilities, integrated with Gaussian’s advanced sampling methods, allow biochemists to simulate membrane proteins in their native lipid environments. This enables the study of conformational changes, ion and small molecule transport across membranes, and the effects of lipid composition on protein function. Such simulations provide valuable insights into cellular processes like signal transduction and drug transport across cell membranes.
Lastly, biochemists can use computational methods to study the dynamics and interactions of large biomolecular assemblies, such as ribosomes, molecular motors, or virus capsids. AlphaPerrin’s coarse-graining tools and multi-scale modeling capabilities, coupled with Gaussian’s force field parameterization, allow for simulations of these complex systems over biologically relevant timescales. These studies can reveal the mechanisms of macromolecular machines, the assembly and disassembly of large complexes, and the cooperative behavior of biomolecules in cellular processes.
Materials Chemists:
In materials science, computational chemistry allows for the prediction of crystal structures and properties. Materials chemists can use algorithms to explore possible crystal packings and predict which structures are most stable under different conditions. They can then calculate properties like band gaps, mechanical strength, and thermal conductivity, guiding the design of new materials with tailored characteristics. AlphaPerrin’s crystal structure prediction tools, integrated with Gaussian’s solid-state calculation capabilities, provide a powerful platform for materials discovery and characterization.
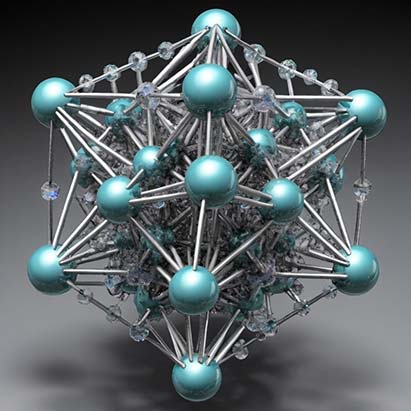
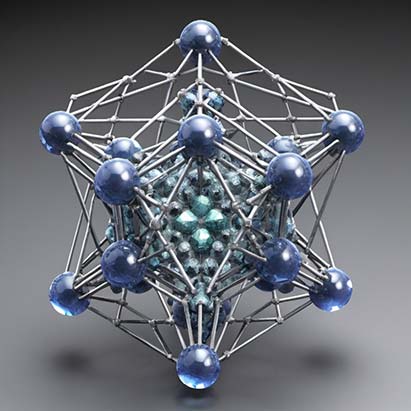
The design of new materials with specific characteristics is a key application of computational methods in materials chemistry. By understanding structure-property relationships, researchers can virtually screen thousands of potential compounds to identify those with desired properties, such as high-temperature superconductors or efficient photovoltaic materials. AlphaPerrin’s high-throughput screening workflows, coupled with Gaussian’s accurate property prediction methods, enable efficient materials design and optimization.
Modeling of defects and impurities in solids is crucial for understanding and controlling material properties. Computational techniques allow materials chemists to study how point defects, dislocations, and grain boundaries affect electronic, optical, and mechanical properties. This is essential for developing materials for applications like semiconductor devices and structural materials. AlphaPerrin’s defect modeling tools, combined with Gaussian’s electronic structure methods, allow for detailed investigation of defect properties in various materials.
Simulation of nano-scale phenomena has become increasingly important as technology pushes into the nanoscale regime. Materials chemists use computational tools to model the unique properties of nanoparticles, quantum dots, and two-dimensional materials. These simulations can predict how size, shape, and surface effects influence material behavior at the nanoscale. AlphaPerrin’s multi-scale modeling capabilities, integrated with Gaussian’s quantum chemistry methods, enable materials chemists to bridge the gap between atomic-scale phenomena and nanoscale properties.
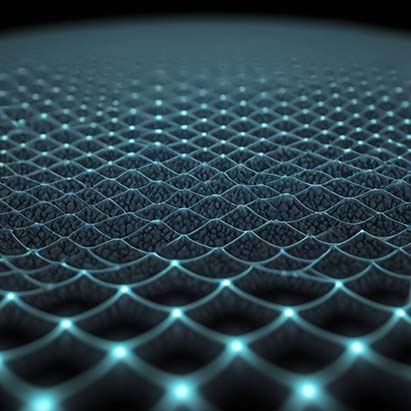
Botanists:
Botanists can employ computational chemistry to model photosynthetic processes at the molecular level. This includes simulating the light-harvesting complexes, electron transport chains, and carbon fixation reactions. By understanding these processes in detail, researchers can work on improving crop yields or developing artificial photosynthetic systems for energy production. AlphaPerrin’s multi-scale modeling capabilities, combined with Gaussian’s excited state calculations, provide a comprehensive toolkit for studying photosynthetic processes.
Studying plant hormone interactions with receptors is another application of in silico tools in botany. Researchers can model how hormones like auxins or cytokinins bind to their receptors and trigger signaling cascades. This can lead to the development of new plant growth regulators or strategies for controlling plant development. AlphaPerrin’s protein-ligand docking tools, integrated with Gaussian’s conformational analysis methods, enable detailed studies of plant hormone-receptor interactions.
Investigating the structure and function of plant enzymes is crucial for understanding plant metabolism. Computational methods allow botanists to model enzymes involved in processes like cellulose synthesis, secondary metabolite production, or stress responses. This can guide efforts to engineer plants with enhanced traits or to produce valuable compounds. AlphaPerrin’s enzyme modeling workflows, coupled with Gaussian’s reaction path analysis tools, provide botanists with a powerful platform for studying plant enzyme function.
Simulating the behavior of plant cell walls provides insights into plant growth and development. Botanists can use computational models to understand how cell wall components interact, how the wall responds to mechanical stresses, and how it is remodeled during growth. This is important for improving crop resilience and developing new plant-based materials. AlphaPerrin’s materials modeling capabilities, combined with Gaussian’s molecular mechanics methods, allow for detailed simulations of plant cell wall structure and dynamics.
Molecular Biologists:
Molecular biologists can benefit from in silico tools by predicting protein-protein interactions. Computational methods can simulate how proteins recognize and bind to each other, forming complexes that carry out cellular functions. This is essential for understanding cellular signaling pathways, protein complex assembly, and designing drugs that target specific protein-protein interactions. AlphaPerrin’s protein-protein docking tools, integrated with Gaussian’s energy decomposition analysis, enable molecular biologists to study the energetics and specificity of protein-protein interactions.
Modeling DNA and RNA structures allows molecular biologists to study how genetic information is stored, accessed, and regulated. Computational tools can predict the three-dimensional structure of nucleic acids, including how they interact with proteins like transcription factors. This is crucial for understanding gene regulation and designing gene-editing tools. AlphaPerrin’s nucleic acid modeling modules, combined with Gaussian’s base-stacking and hydrogen bonding calculations, provide a comprehensive platform for studying DNA and RNA structure and interactions.
Simulating gene expression networks provides a systems-level view of cellular function. Molecular biologists can model how genes are turned on and off in response to various stimuli, and how these changes propagate through the cell. This can reveal emergent properties of biological systems and guide experimental design in fields like synthetic biology. AlphaPerrin’s systems biology tools, coupled with Gaussian’s kinetics calculations, allow for the modeling of complex gene regulatory networks.
Studying molecular recognition processes is fundamental to many aspects of molecular biology. Computational chemistry allows researchers to model how molecules specifically recognize and bind to their targets, whether it’s an antibody binding to an antigen or a small molecule binding to a protein. This has applications in drug design, diagnostic tool development, and understanding the molecular basis of cellular processes. AlphaPerrin’s molecular dynamics and docking tools, integrated with Gaussian’s advanced solvation models, enable detailed studies of molecular recognition events in biological systems.
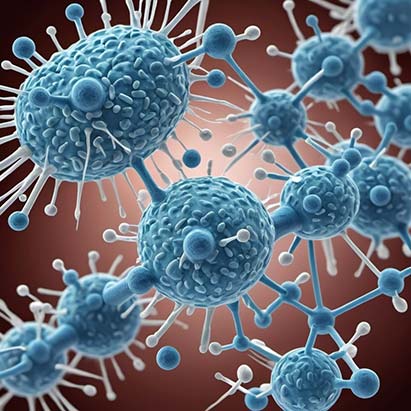
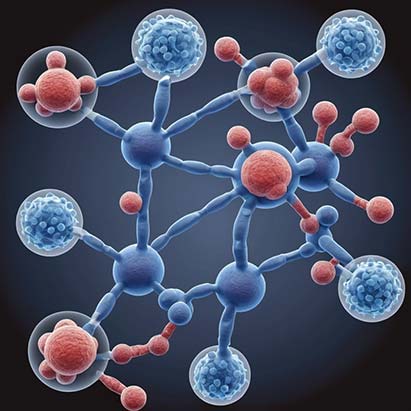
Conclusion
In conclusion, the integration of in silico computational chemistry across these diverse fields offers numerous advantages, including cost-effectiveness, time efficiency, safety, scalability, predictive power, and facilitation of interdisciplinary collaboration. The AlphaPerrin computational chemistry environment, coupled with Gaussian software, provides a powerful and versatile platform for researchers in all these fields to leverage computational methods in their work. As computational power continues to increase and algorithms become more sophisticated, the potential applications of in silico computational chemistry will only expand, playing an increasingly central role in scientific discovery across a wide range of disciplines.
Unlock the future of scientific discovery by starting your own computational chemistry environment. With state-of-the-art tools like Gaussian, you can dive into advanced molecular research, optimize drug candidates, and explore new chemical compounds with unparalleled precision and efficiency. Embrace the power of high-performance computing to accelerate your research, reduce costs, and stay at the forefront of innovation. Start your journey today and transform the way you conduct chemical research. with your own computational chemistry software environment.
Start Today
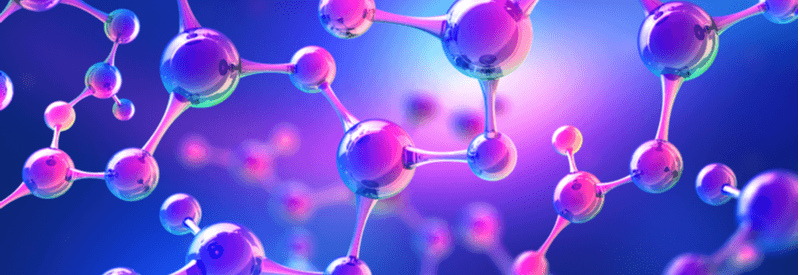
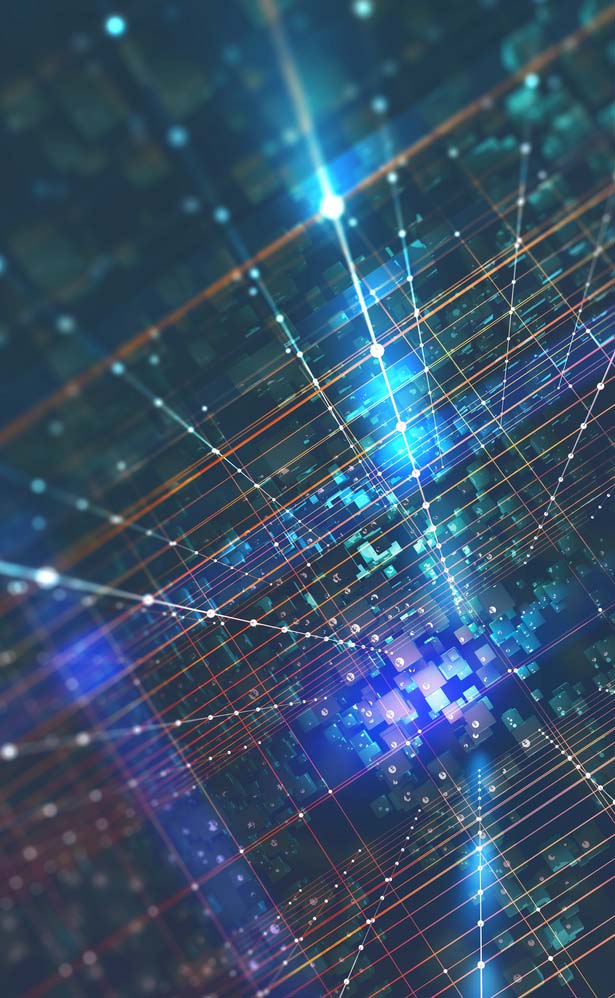
Why In Silico?
Here are six compelling reasons to choose to work with AlphaPerrin for your computational chemistry needs:
Cost Efficiency
Computational chemistry and in silico labs significantly reduce the costs associated with reagents, materials, and physical lab space required for wet labs.
Speed
Simulations and computational models can rapidly screen and optimize drug candidates, accelerating the initial phases of drug development compared to the slower pace of wet lab experiments.
Safety
In silico methods eliminate the risks associated with handling hazardous chemicals and conducting potentially dangerous experiments, ensuring a safer research environment.
Scalability
Computational models can easily be scaled to study larger and more complex molecular systems, which may be challenging or impractical in wet labs.
Predictive Power
Advanced algorithms and simulations provide detailed insights into molecular interactions and predict properties and behaviors that may not be easily observable in wet lab experiments.
Resource Conservation
In silico labs conserve valuable resources, including rare or expensive materials, by allowing extensive testing and optimization in a virtual environment before any physical trials.
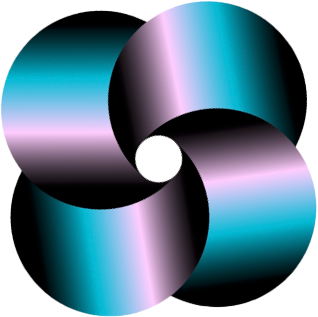